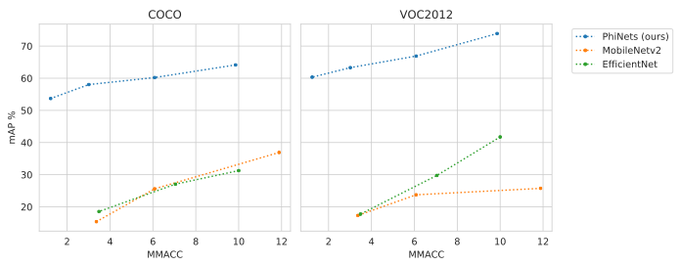
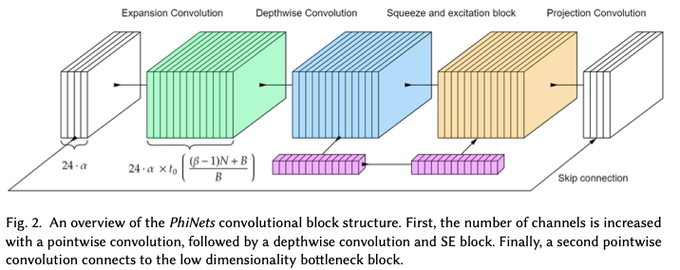
Find the code on Github!
Read the related paper in pre-print on Arxiv
In this work, we present PhiNets, a new scalable backbone optimized for deep-learning-based image processing on resource-constrained platforms. PhiNets are based on inverted residual blocks specifically designed to decouple the computational cost, working memory, and parameter memory, thus exploiting all the available resources.
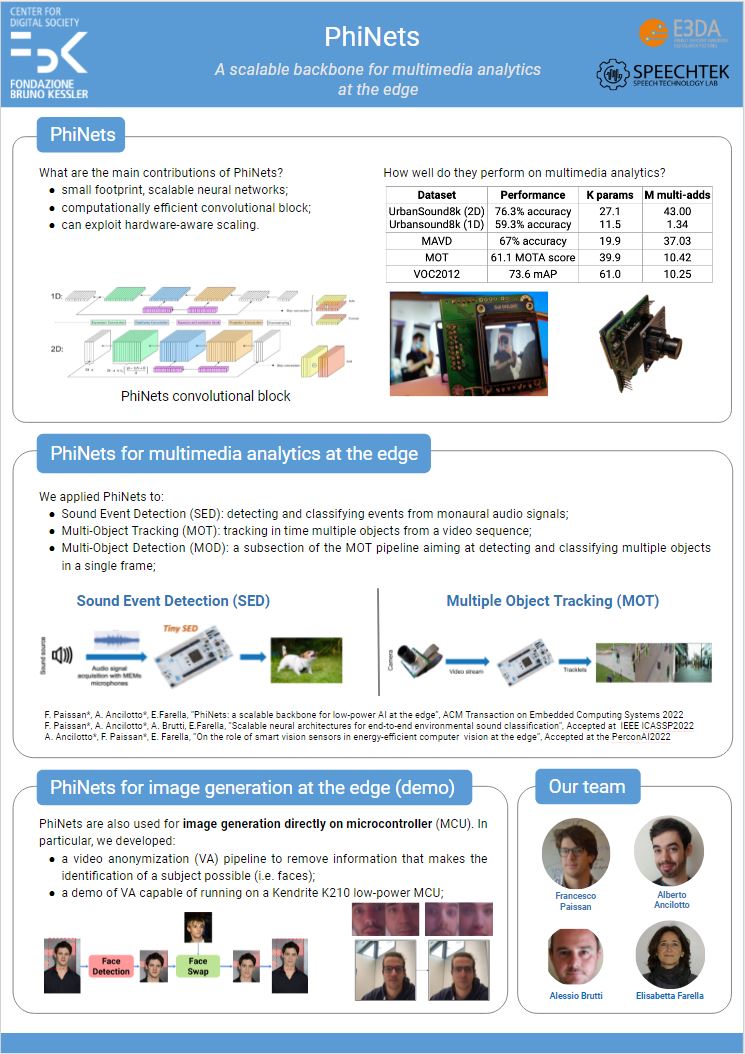